Recent advancements in machine learning have enabled researchers to develop sophisticated computer models capable of predicting everyday events with remarkable accuracy. Traditionally, the focus of these models has been on minimizing prediction errors—essentially, how far off the model's forecasts are from actual outcomes. However, new findings suggest that a more nuanced approach that also accounts for uncertainty can significantly enhance the models' performance and understanding of complex phenomena. This shift in perspective not only challenges established beliefs about prediction errors but also opens new avenues for improving the reliability and applicability of predictive algorithms in real-world scenarios.
The significance of responding to uncertainty lies in its ability to capture the inherent unpredictability of everyday life. In many situations, the actual outcome can be influenced by a multitude of factors, some of which may be unknown or difficult to quantify. By integrating uncertainty into the predictive models, researchers can better account for this complexity, leading to more robust and adaptable systems. For instance, in fields such as weather forecasting, healthcare, and finance, understanding the range of possible outcomes and their probabilities can be just as crucial as pinpointing a single forecast. This approach allows stakeholders to make more informed decisions and prepares them to respond effectively to varying scenarios, thus enhancing overall resilience.
Moreover, the implications of this research extend beyond mere accuracy in predictions. By acknowledging uncertainty, the models can also facilitate better communication of risk and variability to end-users. In practical applications, this means that users can receive not only a prediction but also information about the confidence level associated with that prediction. For example, when predicting the likelihood of rain, a model that incorporates uncertainty might specify a 70% chance of rain with a note that conditions could change. This type of information empowers individuals and organizations to plan accordingly, whether it be carrying an umbrella or adjusting schedules based on projected risks.
In summary, the ongoing exploration of uncertainty in predictive modeling represents a pivotal shift in how researchers and practitioners approach the complexities of forecasting everyday events. By moving beyond a narrow focus on prediction errors and embracing the multifaceted nature of uncertainty, these models can yield deeper insights and foster more effective decision-making. As this research evolves, it is likely to influence a broad array of fields, ultimately enhancing our ability to navigate an unpredictable world with greater confidence and clarity. This transformation not only enriches our understanding of predictive analytics but also underscores the importance of adaptability in the face of uncertainty.
Brain Models Reveal How We Process Life’s Small Surprises - Neuroscience News
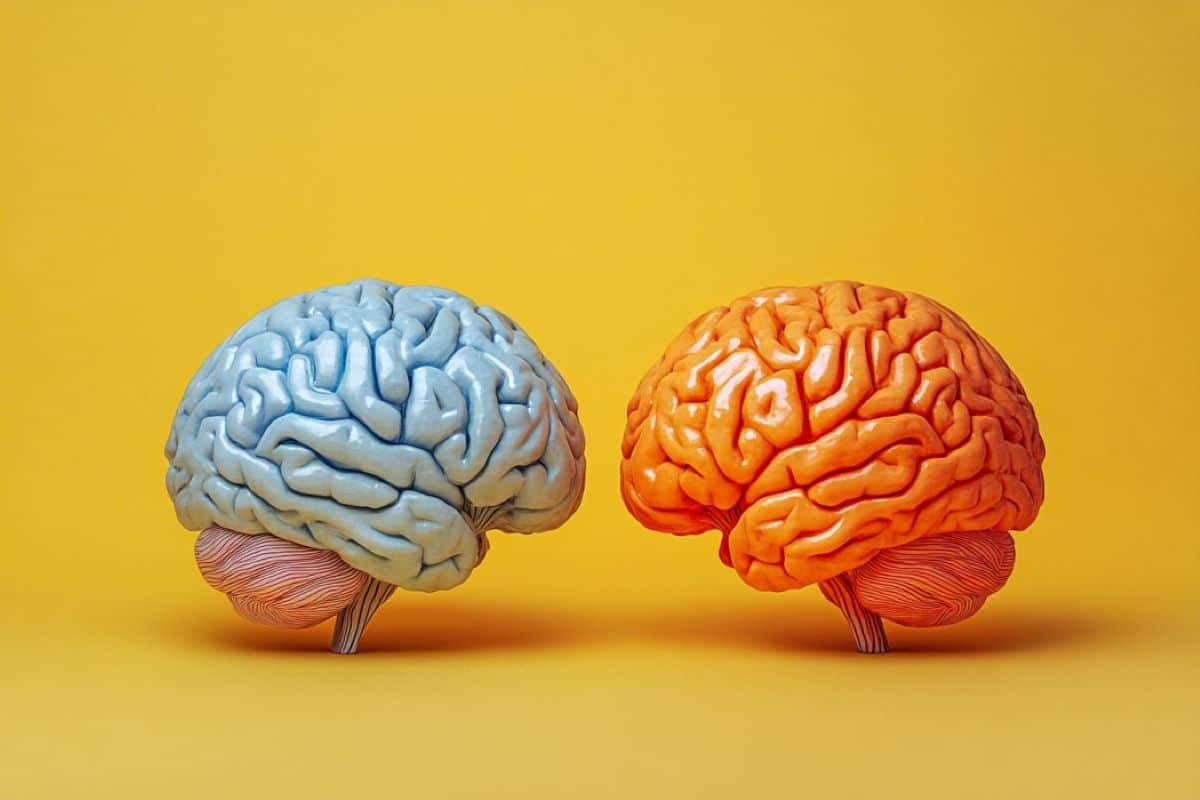